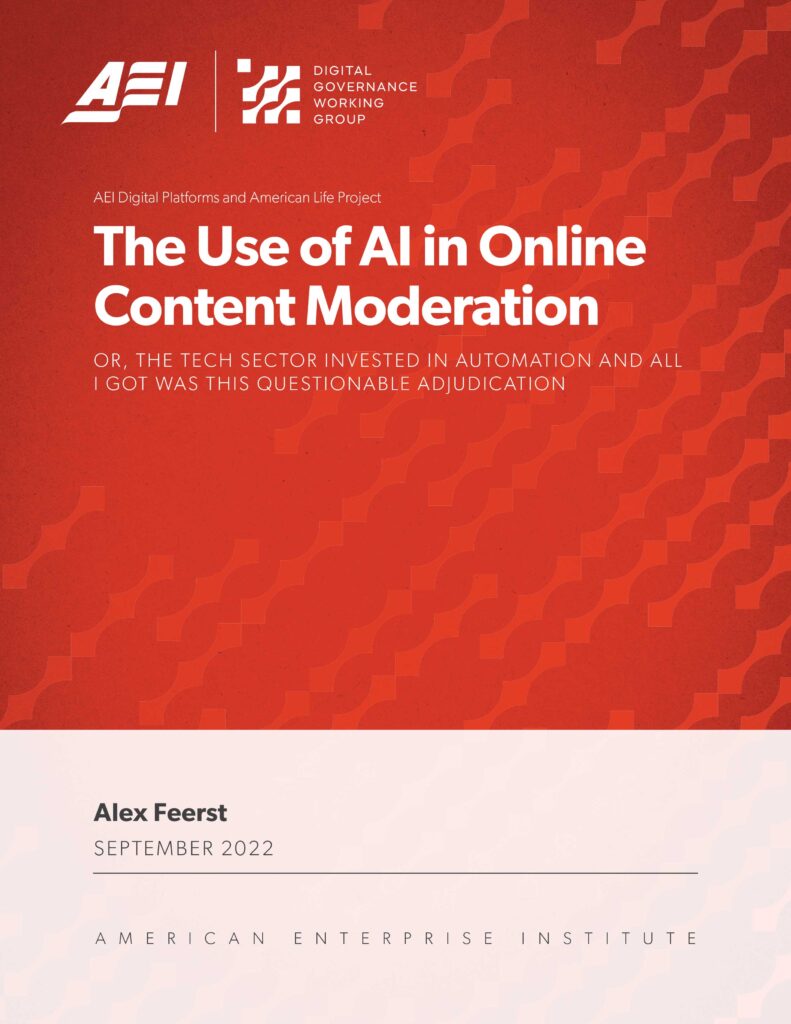
This report explores the role of artificial intelligence (AI) in social media content moderation. It covers: (1) why AI will likely play an increasing role in moderating online expression, (2) why even those with a moderation-minimalist set of starting assumptions will not be able to avoid engaging in moderation and all the challenges and compromises that follow, (3) some examples of what roles AI can play in the moderation process and some of the trade-offs implicit with each type of use, and (4) why expecting too much from AI or failing to attend to its major shortcomings will lead to continuing disappointment and greater injustice.
In March 2021, at one of many congressional hearings on social media, Facebook (now Meta) CEO Mark Zuckerberg reported:
More than 95% of the hate speech that we take down is done by an AI [artificial intelligence] and not by a person. . . . And I think it’s 98 or 99% of the terrorist content that we take down is identified by an AI and not a person.1
Those sound like some high percentages. For some time, tech companies have been pointing to artificial intelligence (AI) as a just-around-the-corner solution to content moderation. (To be fair, some regulators have been asking, “Are we there yet?” for some time too.) Then, suddenly—faster than I expected, at least—those magical robots seemingly got pretty good at classifying the vagaries of human expression. So . . . problem solved? Do we just welcome this era of Pax Machina and get back to posting cat memes and shilling alt coins?
Well, let’s back up first. Why are we using AI to make decisions about online expression? Why would we delegate something this important to nonhumans? What exactly are we using it for? How good is it at it? How would we know if it’s any good at it? How good is good enough? What happens if and when it turns out to be less good at it than we hoped?
The short answer to the question “why AI” is scale—the sheer never-ending vastness of online speech. Scale is the prime mover of online platforms, at least in their current, mainly ad-based form and maybe in all incarnations. It’s impossible to internalize the dynamics of running a digital platform without first spending some serious time just sitting and meditating on the dizzying, sublime amounts of speech we are talking about: 500 million tweets a day comes out to 200 billion tweets each year.2 More than 50 billion photos have been uploaded to Instagram.3 Over 700,000 hours of video are uploaded to YouTube every day.4 I could go on. Expression that would previously have been ephemeral or limited in reach under the existing laws of nature and pre-digital publishing economics can now proliferate and move around the world. It turns out that, given the chance, we really like to hear ourselves talk.
This should come as no surprise because most of us (those without printing presses or newspaper columns or press secretaries) have waited for most of history without access to broad, cheap, and non-ephemeral distribution and had a lot to say. Compared to previous options like writing a strongly worded letter to the editor or buying some billboards, it’s a great time for people to communicate with others and try to gain renown or paying work from it. If you’re a fan of voluminous and diverse expression—especially by people who previously didn’t have presumed access to broad distribution channels—this is a win (which has brought with it new dangers, like doxing, swatting, and general harassment, which are not a win).
Given the recent fixation on social media and its harms, it’s easy to forget what things were like before. Even with warts and all, the era of digital platforms brought a profoundly equalizing shift in communication (built, admittedly, on an un-equalizing shift in surveillance and harassment). The internet is eternal amateur hour in the best and worst ways, and the implications of that, for learning more about ourselves and each other, are still staggering (though none of us may ever know as much as the companies or governments that do the tracking and hold the data). But at the same time: 500 million tweets and 720,000 hours of video a day? A shift in the nature and dynamics of human communication that big is going to have multiple waves of serious effects.
So, we’ve been on a self-expression bender for well over a decade. So what? Why would anyone want to ruin this with content moderation, whether done by AI or just people? Why not celebrate what we have and let the people or the market or God sort it out? Each reader can apply their reason to what they read and see and be tolerant of things they disagree with, people will develop a thick skin for unkind words, and the invisible pipes will direct the supply of smart thoughts to where matching demand exists. No gods, no moderators.
The question of whether, what, and how much to moderate—and the pragmatic and philosophical implications of those choices—is a giant can of worms that deserves its own book. For our purposes, let’s start with assuming we want to do no more than minimum viable moderation, meaning a service provider should intervene only where it would be illegal not to. When we start trying to implement this minimalist, only-illegal-things-come-down playbook, however, we quickly come across a key question: Illegal according to whom?
Courts don’t weigh in on most human conduct or expression, and that’s a good thing. Taking every harmful online post to trial would be more expensive and time-consuming than any of us would care to imagine. Some real-world minimalists (such as certain Latin American legislatures, the Indian Supreme Court, and the Electronic Frontier Foundation) would, say, remove an online post for nothing short of a court order, using the inconvenience and expense of a trial to protect as much expression as possible. Now that’s free speech. Nowadays this is a pretty rare position for practical reasons that become obvious once you try to enforce it.
Understanding why even minimum viable content moderation is hard requires getting serious about two interrelated concepts: human subjectivity and how much of the adjudication happening in the world is extrajudicial—meaning it happens out of court.
Very few types of content or conduct are clearly illegal. For example, child sexual abuse material is obviously illegal; the hard part is identifying it without ruining people’s lives when you find false positives. But for most types of content, it’s not so clear-cut. Figure 1 shows a useful diagram Stanford Law School Professor Daphne Keller made illustrating the problem.
In many cases, determining illegality is a matter of applying a highly subjective set of factors using good old-fashioned legal judgment, predictions about how a court might rule, and risk management. In the United States, for example, there is no statutory protection from secondary liability for trademark infringement. So, say someone uses your service to post something, and someone else accuses that person of trademark infringement for, say, promoting or selling counterfeit goods. Is it illegal for you to host that original post? Well, it depends. You’d need to conduct a reasonable investigation about the facts and then decide if the material is infringing. In other words, you would do what corporate lawyers do everywhere, which is evaluate the legal risk and then do your best to reduce the risk to your company based on cost-benefit analysis.
“It depends” is one part of why people who think that it should be easy to just moderate clearly illegal content are wrong. Most decisions about what online speech is “illegal” are extrajudicial, so it’s a guessing game, and in most cases you’ll never know what a court would say. For a given online post, the poster, the person mentioned in or affected by the post, and the company providing the service may each arrive at different views on the post’s legality. In fact, it’s a virtual certainty they are going to disagree. Each will apply the law (as they understand it) to the facts (as they see them) and arrive at different conclusions. As the Dude would say, when we’re not in court, what is “illegal” or “clearly illegal” is just, like, your opinion, man.
At this point, the company steps in and makes a decision. Why? Because someone has to. Because it runs the service and has the power to make decisions and take actions and is ultimately answerable to its shareholders to keep risks low and profits high. Because it doesn’t like when users complain on social media, reporters write stories about abuse on its platform, and legislators score points off them to rally the base. It’s not a court, but before long, it will likely adopt optics and procedures resembling a court’s to make decisions that have greater actual and apparent legitimacy, which then allows them to be applied at scale. The service engages in an act of private adjudication. And now we’re off to the content-moderation races. Even this minimalist strategy will never not be controversial because people are going to disagree about what is illegal and wonder who decided that the company gets to decide what’s what.
This inevitable disagreement among potentially infinite people about what is what is not a sideshow. It’s the often and problematically overlooked main event when we talk about AI content moderation. If I have one message, it’s this: Don’t confuse a subjectivity problem for an accuracy problem, especially when you’re using automation technology.
Source: Courtesy of Daphne Keller (platform regulation director, Stanford Cyber Policy Center).
We can ask, for example, how accurate a given AI is at classifying whether something is a dog or a muffin, and we can probably learn how to improve its accuracy rate. That’s because in such cases, humans can more or less agree on a ground truth that the AI conforms with or deviates from. But with many kinds of expression—like “is this hate speech?”—it’s not a question of accurate or not. There will be as many opinions as there are people. Three well-meaning civic groups will agree on four different definitions of hate speech.
You might experience this as an accuracy problem (“companies are inconsistent in taking down content” or “why is this fool so stubbornly wrong about what is and isn’t hate speech”), but it’s really a subjectivity problem. We’re stuck in our own skin and universalizing our judgment.
If the things we’re doing are controversial among humans and it’s not even clear that humans judge them consistently, then using AI is not going to help. It’s just going to allow you to achieve the same controversial outcomes more quickly and in greater volume. In other words, if you can’t get 50 humans to agree on whether a particular post violates content rules, whether that content rule is well formulated, or whether that rule should exist, then why would automating this process help? You might train an AI to get pretty good at identifying pastries, but no technology will get people to agree on what is and isn’t hate speech and whether it should be permitted in a given place. This is why, even if AI can help, it alone probably can’t help as much as we’d like. So if we increase pressure on companies to promise to fairly remove only the really offensive and illegal content, we’re setting everyone up for disappointment later.
There’s plenty more to say about rule sets and trying to enforce them, but I will leave it here. As governments increasingly tinker with the machinery of online censorship, we can expect more laws to comply with. So regardless of whether you are a “bring me a court order in triplicate or it stays up” kind of person or a “let’s make a set of speech rules more complicated than zoning in San Francisco” type, at some point you have to move from figuring out your philosophy to drafting your rules and enforcing them. You need to translate the rule set into action. And as fascinating as free speech doctrine is, running a social media company is a practical business, not an academic exercise. You’re not a hydrologist; you’re a plumber. You can’t spend your days entranced by the properties of water; your job is to make the drains and faucets work.
The era of mega platforms brought massive demand for private adjudications of speech-related disputes. The unit economics of court adjudications with unabridged, individualized fact-finding and due process is out of the question. It would bankrupt us all. One possible conclusion here is that once we have fully and honestly internalized the social and economic costs of social media in its current form, we would see that it is simply too expensive to exist. But, if we assume that platforms must continue to exist (an assumption some would say we should abandon ASAP), then without tossing aside due process and fairness, but also without sentimentality about putting a price on dispute resolution given how much work we have to do, we have to figure out how to bang out hundreds of thousands of daily speech adjudications on a budget. And that brings us back to AI.
Imagine you’re the proud new owner of an online platform. Congratulations! You have a lot of content to review and decisions to make. So who’s going to do it? For now you really only have two and a half options—people, machines, or some combination thereof. Let’s go through some of the variations. Remember, we’re on a budget.
If you use people, they can be paid or unpaid. If paid, they can be employees or contractors, located in higher or lower cost-of-living places in the United States or potentially even lower-cost places overseas. You can pay them well or as little as possible, train them a lot or not so much. You can offer them career advancement, benefits, free snacks, and counseling or not. Maybe some of these variables will lead to reductions in volume or quality, but possibly not linearly or even predictably.
If unpaid, people might choose to do this work for a range of reasons, such as because they really care about the community they belong to, gain social capital for it, enjoy the feeling of power or being an insider, have nothing better to do, take a prurient interest in extremes of human behavior, or hope to get crypto tokens someday—or some combination of the above. Depending on the nature of your service and even given the endless human capacity for unpredictable behavior, there are probably limits to how much free moderation work people will do (or how much labor law will allow), and it’s harder to control quality and training. (Do you want volunteers handling your frontline risk management?) Even with unpaid volunteers, coordinating them to follow some broader set of meta-rules is not necessarily easy or cheap.
With any combination of the above, at scale, it’s going to add up. Ten thousand employees here, ten thousand ergonomic mouse pads there, and pretty soon we’re talking about real money. This is what the cost of running a platform looks like, once you’ve internalized the harmful and inexorable externalities we’ve learned about the hard way over the past decade.
Software tools of the AI and non-AI variety can help human moderators. It can make them more efficient, more accurate (whatever that may mean), more perceptive, and less likely to suffer trauma from what they see. At this scale, AI software that is reasonably accurate would reduce the need for human moderators by a lot. If you can save a few billion dollars a year on moderation, it makes running a platform go from a terrible idea to a possible money maker. This is why highly accurate AI has been both the holy grail and the assumed inevitable end point of content moderation.
So, what do we talk about when we talk about AI for content moderation? AI—computer systems trained on large datasets and oriented toward particular problem-solving tasks in ways intended to mimic human intelligence—can be used for a range of tasks associated with moderation (though notably, competently labeled large datasets don’t exist for many regions, cultures, and languages). Which tasks has it been good for so far? Which ones do tech companies plan to use it for? And what are the implications of deciding to use AI rather than humans for a particular task or type of task?
Let’s take a closer look at Zuckerberg’s statements. He said that more than 95 percent of hate speech is taken down by AI, which I take to mean that AI software is making an automated adjudication (deemed a post to be hate speech in violation of a content policy) and then executing an automated action (the takedown). He went on to say at least 98 percent of terrorist content is identified by AI—presumably leading to subsequent steps, such as adjudication, choice of remedy, and enforcement action likely taken by a human acting downstream based on the AI identification.
One type of moderation task AI should be good for is recognition (or discrimination)—in other words, classifying inputs (thought it often isn’t for a variety of reasons). For example, AI should be able to reliably say “this image is/isn’t a dog” or “this image is/isn’t a human female nipple.” If your platform has a rule against nipples (leaving aside the sexism and prudery underlying such a rule), then AI image recognition like this can be useful. Now, the rule may actually be something more complex, like “no nipples, except in a breastfeeding context,” or “nipples OK, except in a sexualized context.” But let’s keep it simple for now.
This makes sense on a high level. Like any kind of adjudication, moderation begins with observing something (content or conduct manifested in some observable online form), applying some rule to the observed fact, engaging in analysis, and then deciding what to do about it. There are lots of ways that AI can be and is used at the early stages of this process. So, even leaving aside the hard work of analysis and remedy, AI used for detection and classification can do a lot to sort content for human review. This lets you do things like channel likely extremist content to reviewers with expertise in that area, while sending potential nipples to a different set of specialists, working at a different price point.
The inputs, context, and goal can vary depending on the type of content you’re dealing with, what kind of harm you’re trying to mitigate, or what type of rule you’re trying to enforce. Let’s say, for example, you are using a model to detect human nipples in images. What you do with the outputs is a separate question. If an image is flagged by the AI, you can send it to a human for confirmation and then have the human make an adjudication and decide what action to take (say, block, allow, or send a warning). Or you could have the AI automatically block the image if the model is more than, say, 95 percent confident that it is indeed a nipple. Or you could aim for some other contextual constraint: For example, if the model is more than 95 percent confident that the image contains a human nipple and more than 80 percent confident that there is no baby in the picture, then the AI should block the image (or surface it to a human for review and decision).
Expanding the frame, detection can involve not just content but other forms of data or metadata. For example, if you are tracking a disinformation campaign, yes, the content may matter, but so do extrinsic factors, like which accounts appear to be posting or attempting to amplify this content, how long the accounts have existed, where the users appear to be logging in from, whether they are connected to known past incidents or campaigns, whether the profile picture is an image found elsewhere and associated with particular groups, and whether there is a pattern in the way groups are connected to each other. There are a lot of data that can be interpreted as strong or weak signals that in the aggregate can suggest an explanation for what is going on and what to do in response. To return to one of Zuckerberg’s examples, AI trained on terrorist content might be helpful with a range of fully and semiautomated tasks, such as sweeping the network for user-uploaded copies (or slightly altered copies) of a mass shooting to surface for human review, with the suggested parallel actions of leaving them up in the US (where it is legal) but blocking them in Australia (where it is not).
The problems with using AI are many. On June 16, 2022, the Federal Trade Commission issued a report to Congress about some of them.5 For starters, AI is biased. There is ample research on the many biases embedded in AI, but they shouldn’t be surprising; AI is designed and trained by humans. It reflects, encodes, and enshrines existing power relationships and inequalities. Depending on things like whether the training is supervised or unsupervised, a model is either trained on labels made by imperfect humans or, in more complex and indirect ways, ingests data reflecting the structures of current human experience and detects highly correlated elements. It’s hard to overstate how huge a problem this is. If we’re going to delegate detection, much less decision-making, to AI, we risk entrenching and multiplying decisions that are fundamentally unfair and, worse, biased against less powerful people—in other words, the people who arguably most need online platforms.
There are also dignity issues. Many people just don’t like the idea of a machine making decisions about something that feels as irreducibly human as speech. That may change at some point, but who knows when and whether that change would be good. In my view, one reason so many laws in this area address the ability to appeal decisions is the tacit or explicit discomfort with automated adjudication without an explicit mechanism for triggering human review.
A related issue is what AI people call “explainability.” As laws (primarily in Europe for now, but likely coming soon to a jurisdiction near you) call for increased transparency in content moderation, privacy, algorithmic recommendation, and the use of AI, this issue will present a challenge. AI outputs can be hard to explain. In some cases, even the creators or managers of a particular product are no longer sure why it is functioning a particular way. It’s not like the formula to Coca-Cola; it’s constantly evolving. Requirements to “disclose the algorithm” may not help much if it means that companies will simply post a bunch of not especially meaningful code.
There is one more issue. Content moderation is a dynamic and adversarial game. In cases where there are bad guys acting intentionally to achieve a particular goal—such as a disinformation campaign aimed at discrediting a candidate, damaging a company, or dividing a nation—they can use evolving AI tools to evade or defeat your AI tools. So, even as AI tools improve, the contexts they operate in will continue to evolve.
There will never be “set it and forget it” technologies for these issues. At best, it’s possible to imagine a state of dynamic equilibrium—eternal cops and robbers. It’s also possible to imagine an outright “dead internet” where us humans struggle to find each other in a garbage internet of mostly AI-generated content and bots interacting with each other.
Given the current scale of online speech and the current mess of hybrid human-tech problems, AI will no doubt increase in sophistication (which should not be mistaken for an increase in quality), and its role in determining what social media users view, post, and engage with will increase and transform. There are good reasons to be cautious about how reliant we become on AI and how quickly. Where it makes most sense to ensure humans remain in the loop, we should take care to do so, even when it might be tempting to cut us out.
We must not get into the habit of viewing AI as a simple solution to society’s ills, especially as AI applications get more impressive. This is because, at its core, illegal and harmful online expression is not a problem to be solved, and the issues it crystallizes are not limited to online platforms, though platforms may provide a novel and visible manifestation of deeper social issues. Harmful online expression is not a temporary problem; it’s a pervasive condition (the human condition) to be managed over time along with other social values, challenges, and institutions. There will be good days and bad days, tragedies averted and ones that evade detection until too late, adversaries who get the jump on us and ones who years later turn out to be less competent than advertised, harmful social movements that clearly metastasize in front of our eyes and ones that (initially at least) just look weird and random. Myriad imagined and unimagined crises are yet to come.
There’s no silver bullet for any of this, but given the path we’re going down, some combination of humans and AI will play an increasing role in shaping our day-to-day experience of the vast amount of online expression, which, if I know us, is not going to subside anytime soon. So, if we proceed this way—and it looks like we will—I suggest that whenever you see “AI content moderation,” try mentally substituting “machines efficiently applying all our biases, one by one, then all at once, then stacked and multiplied.” And if the question is “Will this trend help or harm the state of online expression?” then the answer is “yes.”
Alex Feerst leads Murmuration Labs, which works with leading tech companies and Web3 projects to develop online trust and safety products, policies, and operations. He was previously head of legal and head of trust and safety at Medium and general counsel at Neuralink. In 2021, he cofounded the Digital Trust & Safety Partnership, the first industry-led initiative to establish best practices for advancing and assessing online safety. He serves on the boards of the Responsible Data Economy Foundation and the MobileCoin Foundation and on the editorial board of the Journal of Online Trust and Safety.